The ceaseless whirl of innovation in the technology sphere brings us to three compelling topics that bear discussing: the shifting currents of the AI landscape in industry, the stratification of AI proficiency into a ten-step ladder, and the intricacies of the LLM fine-tuning initiative. Firstly, we delve into the AI industrial panorama, not attempting an exhaustive analysis, but providing more a snapshot of the ongoing developments, like an impressionist painting allowing the contours to form shifts in AI's adoption and adaptation. Secondly, we lay out the intriguing contours of the AI proficiency landscape. In a continuously evolving digital environment, sifting through the ten levels of expertise ranging from novices up to strategic leaders uncovers an essential rubric for understanding the application and integration of AI within various sectors. Lastly, the spotlight turns to the specific aspects of the LLM fine tuning initiative - a path to refining and honing language learning models.
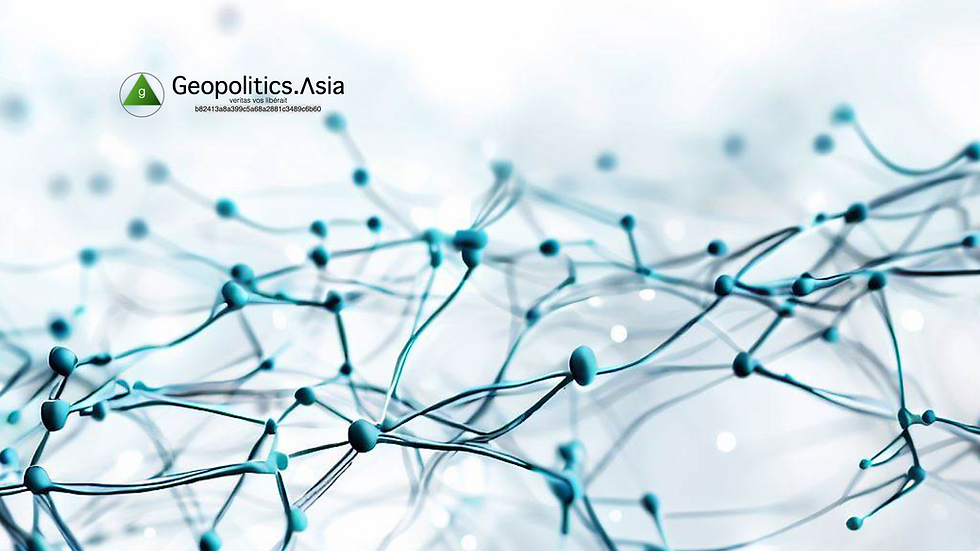
The Foundation
Diving into the "AI Industrial Snapshot", the appetite for AI construction emanates from diverse sources, a mix of technical prowess and the charisma of several influential figures in the field. Towering among them are the celebrated 'big three': Prof Yann LeCun, Geoffrey Hinton, and Yoshua Bengio. These thought leaders were jointly graced with the Turing Award in 2018, the proverbial Nobel in computing, for their landmark breakthroughs in AI. LeCun, presiding presently as the Chief AI Scientist at Meta, the reworked identity of the Facebook group, casts a long shadow over the industry.
Yet, to truly explore the dawn of AI, our gaze must shift to DARPA, the Defense Advanced Research Projects Agency. This organization was not just the harbinger of the internet with its progenitor ARPANET, but also the champion of nascent but pivotal tools such as UNIX and TCP/IP.
To truly appreciate DARPA's pivotal role, we must rewind history and scrutinize the agency's origins during the chilled climate of the Cold War. Born out of the 'Sputnik shock'—the Soviet Union's game-changing launch of the first artificial satellite—DARPA was the United States’ tactical answer to secure its own technological dominance. This era-defining event catalysed the invention of groundbreaking technologies, including Advanced Research Projects Agency Network (ARPANET.)
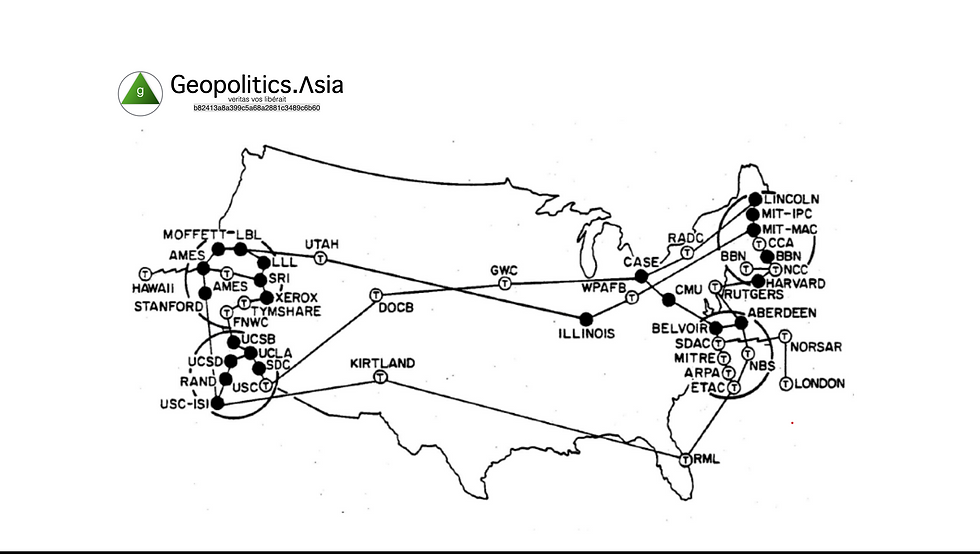
ARPANET access points in the 1970s: [source]
Importantly, ARPANET's development was intimately aligned with a stark reality of the time: the potential doomsday scenario of a nuclear war. The system was crafted to withstand traditional communication breakdowns that could occur in such catastrophic circumstances. The masterstroke was its automatic rerouting capability, ensuring that ARPANET could continue functioning seamlessly in the face of a nuclear onslaught, offering a reliable option that could outlive and outperform conventional telephone lines.
UNIX and TCP/IP, the unsung heroes of the digital age, were conceived, nurtured, and polished under DARPA's umbrella. UNIX, a versatile and multiuser operating system, and TCP/IP, the suite of communication protocols used to interlink network devices on the internet, closed the chasm between diverse computer systems.
Cognitive Enhancement Project
Following the cessation of the Cold War, the United States grappled with an evolving dilemma— the Global War on Terror (GWOT). In this new epoch, the dread of nuclear conflict with superpowers like the Soviet Union was overshadowed by sporadic, unpredictable acts of terrorism, such as the September 11 attacks orchestrated by al-Qaeda.
In the absence of tangible warfare, the US embarked on the modernization of its military forces, keen to become more strategic and intelligent. Thus, the doors were opened wide to AI and automation, tools proficient in generating nuanced assessments and simulations, and unnervingly close to "making decisions".
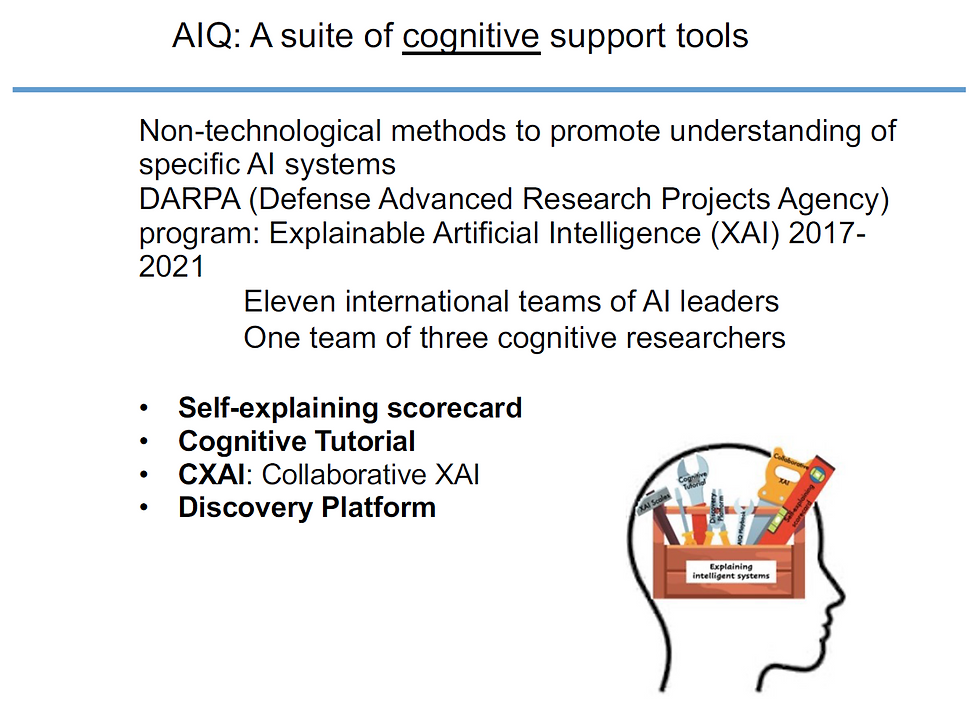
This raises a pertinent query: to what extent will AI feature in strategic decision-making processes? This centuries-old discussion, often termed "expert systems", garners renewed interest in the context of AI. DARPA, maintaining its trailblazing role, aspires to incorporate not entirely machine-led decisions, but strategic, machine-generated advisories.
Many programs under the US Department of Defense, responding to the exigency of robust information analysis and decision-making capabilities in intricate and emerging issues, are echoing this approach. These include the Combating Terrorism Technical Support Office, the Air Force’s initiative towards new optimization and computational paradigms for complex engineering systems design under uncertainty, the Navy’s future computing and information environment, and the Army’s core competency programs in decision sciences and human-system integration. An across-the-board requirement is emerging for operational systems and working methodologies that are adaptive, resilient, and equipped to confront unpredictable and shifting scenarios.
Gary L. Klein (see interview podcast, better not to confuse with Gary A. Klein), a senior principal scientist in cognitive science and artificial intelligence at the MITRE Corporation’s Information Technology Center, has contributed numerous publications on enhancing decision-makers’ option awareness in complex decision environments. Active as a technical advisor and consultant to numerous government programs on sociocultural sense-making and decision-making under uncertainty, Klein currently leads MITRE's advisory role to DARPA’s Next Generation Social Science (NGS2) program. The goal is to foster large-scale experimental research to grasp the emergent properties of human social systems. Additionally, Klein advises the U.S. Office of the Secretary of Defense’s Human Systems Community of Interest in devising a science and technology roadmap for the deputy assistant secretary of defense for research and engineering.
The Social Radar
Understanding societal undercurrents isn't limited to boardrooms or strategic think tanks, but has also captured the attention of the U.S. Air Force. They're developing a concept known as "Social Radar," a non-traditional radar that isn't focused on physical measurements, but rather, tuned in to societal dynamics.
This virtual sensor encompasses myriad technologies and disciplines aiming to measure and predict societal vigor, part of a more extensive Pentagon initiative. This effort seeks to comprehend the sociocultural facets of warfare, an arena known to test even the most stalwart Defense Department veterans. An essential initial step channels into social media networks like Twitter, aiming to discern patterns of social distress.
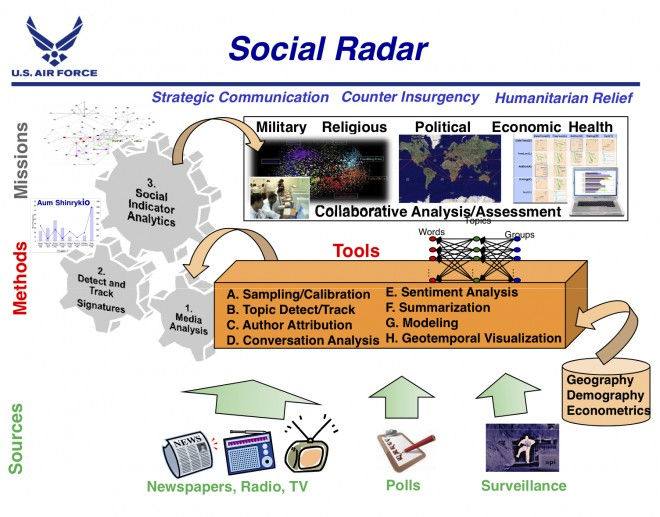
As the Air Force's chief scientist Mark Maybury asserts, their role has transformed from a traditional focus on Intelligence Surveillance and Reconnaissance (ISR) to forecasting enemy movements. This new mandate necessitates human behavior understanding, encapsulating individual motivations, actions and responses.
Maybury, a specialist in artificial intelligence and language processing with decades-long intermittent military association, has found himself increasingly enmeshed in what he refers to as the "human domain" of warfare, particularly as prolonged counterinsurgency efforts in places like Iraq and Afghanistan continue.
In summary, the U.S. Air Force's exploration of the Social Radar highlights another aspect of the military modernization drive, illuminating how AI is being strategically employed not only for traditional surveillance but also a wider and more nuanced understanding of adversary behavior within a societal context.
Culture-on-a-chip
While our PulsarWave project echoes thematic similarities with Maybury's "Social Radar", the objectives diverge rather distinctly. At SIU, a civilian think tank, PulsarWave delves into the zeitgeist of current affairs, aiming to automate strategic scenario planning and PESTLE report generation supporting policy analysis and global risk assessment. The results are tailored to inform policy planning and portfolio investment strategies.
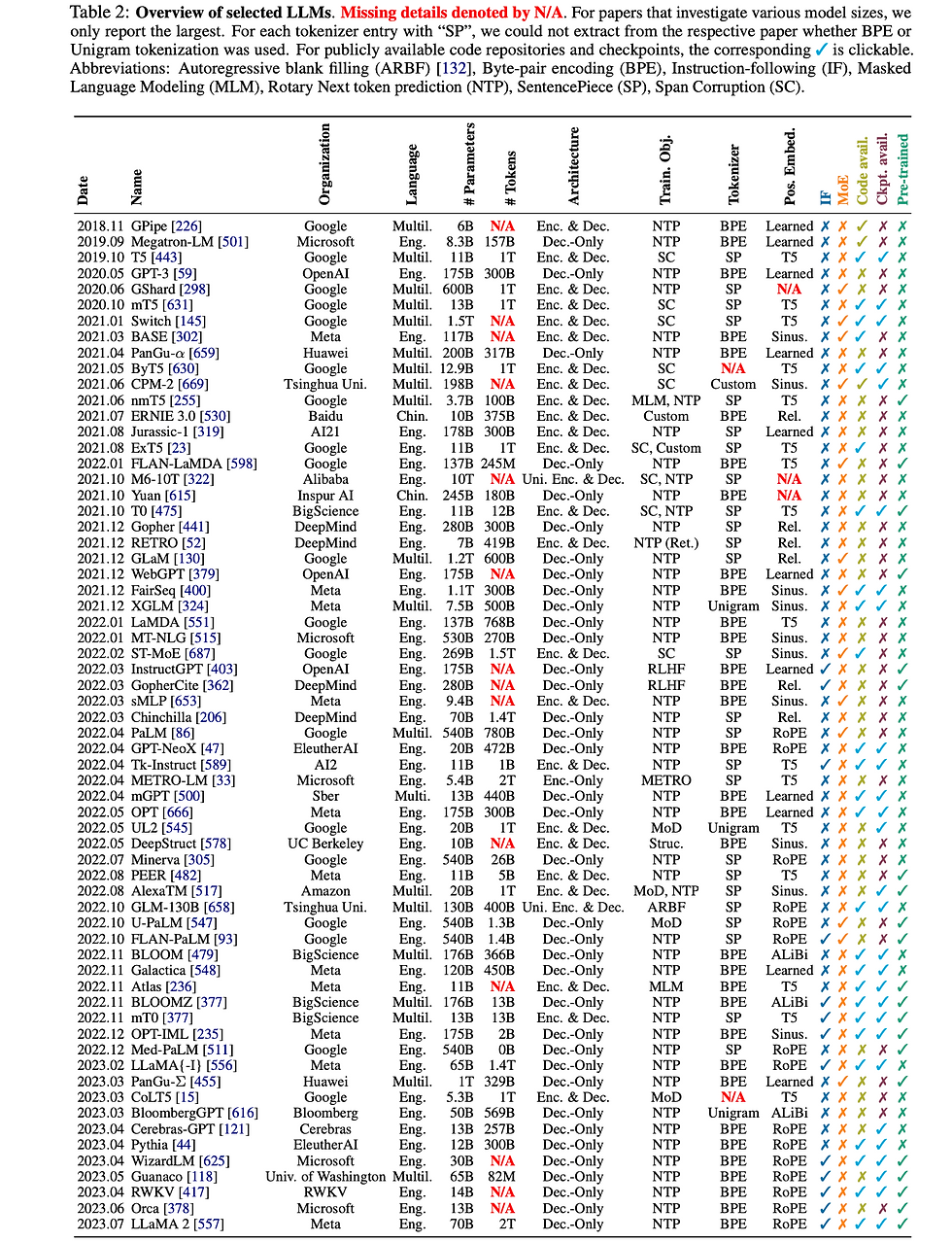
Contemporary LLM's landscape, source: [Challenges and Applications of Large Language Models]
However, the lessons gleaned from DARPA's NGS2 initiative - also known as its counterpart, "the ground truth" - and Maybury's "Social Radar" project serve as valuable compass points for our research program's trajectory. Our goal is to develop functional and operational "cognitive support tools" aimed at amplifying human cognitive capacities in the context of social science.
Inspired by missions like the "culture-on-a-chip" program, which attempts to quantify the formations and collective identities of cultural groups, we aim to introduce a quantifiable approach to social science. This lens varies greatly from endeavors focused on concocting an engaging chatbot or creating aesthetically pleasing images or music through AI. While these are certainly pivotal undertakings, especially in the realm of the entertainment industry, as well as the unintended consequences, our focal point remains clear: to harness AI's potential in augmenting human cognition.


Therefore, as we navigate the current shallows and depths of AI developments, our evaluation leverages a singular criterion: the extent to which AI can enhance our cognitive faculties. This will form the compass by which we assess the journey and progress in the AI landscape.
Our Assessment
In our appraisal of the latest Language Learning Models (LLMs), we project Google's PaLM 2 as the frontrunner. OpenAI's GPT-4 and Antropic's Claude 2 share the runner-up spot, while Meta's LLaMa 2 finds itself in third place—a shift from our previous analysis and some claims made on AI competition boards.
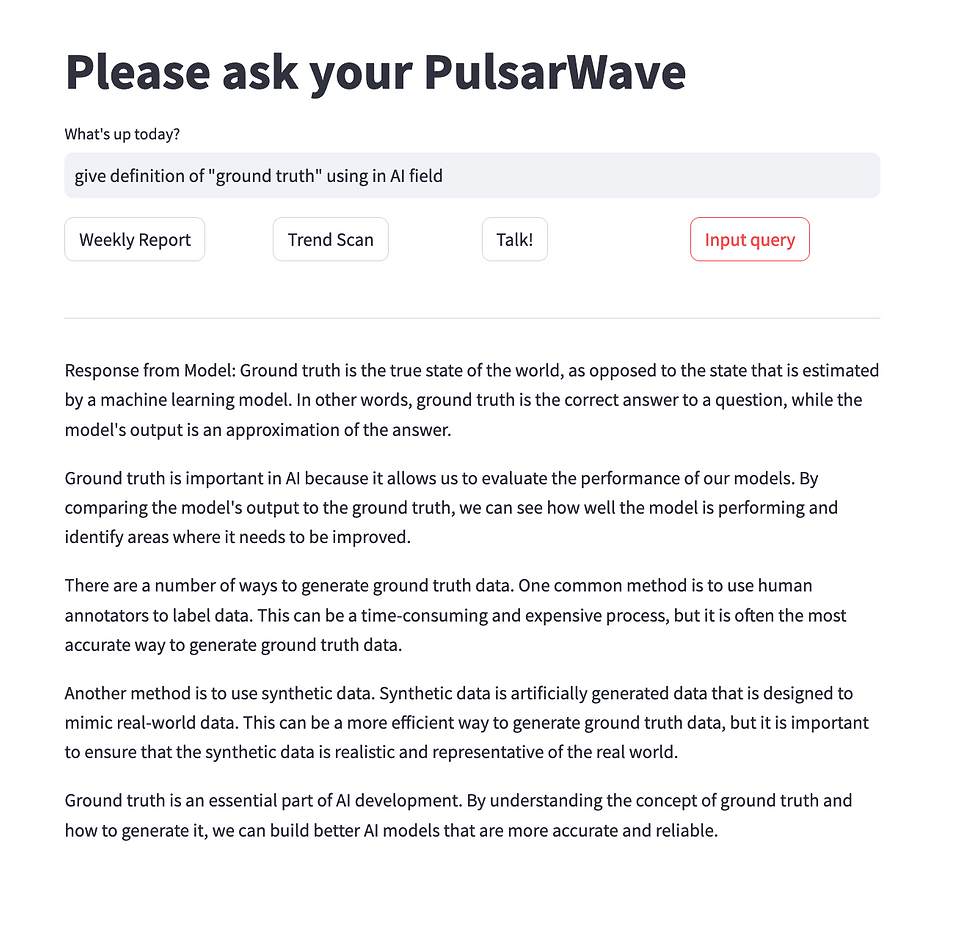
Our observation spots a slight dip in the performance of GPT-4, the cause of which remains elusive. Meanwhile, PaLM 2 has consistently demonstrated exceptional analytical and summarization skills—precise, forthright, and timely, akin to the credo of Ken Thompson: "What is the problem? No message, there will be a message once there is a problem."
Impressively, if supplied with accurate data, PaLM 2 excels, albeit with a propensity for 'hallucination' when fed ambiguous data. Yet, GPT-4 and Claude 2 have shown a slightly nuanced ability to sidestep such 'hallucinations' under similar circumstances. We note that GPT-4 has occasionally created hallucinations, possibly as a result of issues with its training data and a leaning towards a 'chatting' approach, a trait notably subtler in GPT-4 API than its ChatGPT pro counterpart.
Meta's LLaMa 2, despite producing occasionally unsatisfactory output due to its own instances of hallucination, shows promise due to its open-source foundation and consequent adaptability, a trait less observed in GPT-4 and Claude 2 who tend to self-correct within a few iterations.
As we transition to the next section, we will delve into potential strategies for developing curated curricula to hone AI expertise within human resources.
A 10-Level Curriculum for AI Learning
The proposed AI learning curriculum, with suggested reading material, consists of the following ten levels:
Level 1: Basic Programming Knowledge: At this stage, learners need to focus on understanding a programming language, preferably Python. Some of the key areas covered are basic data structures and algorithms.
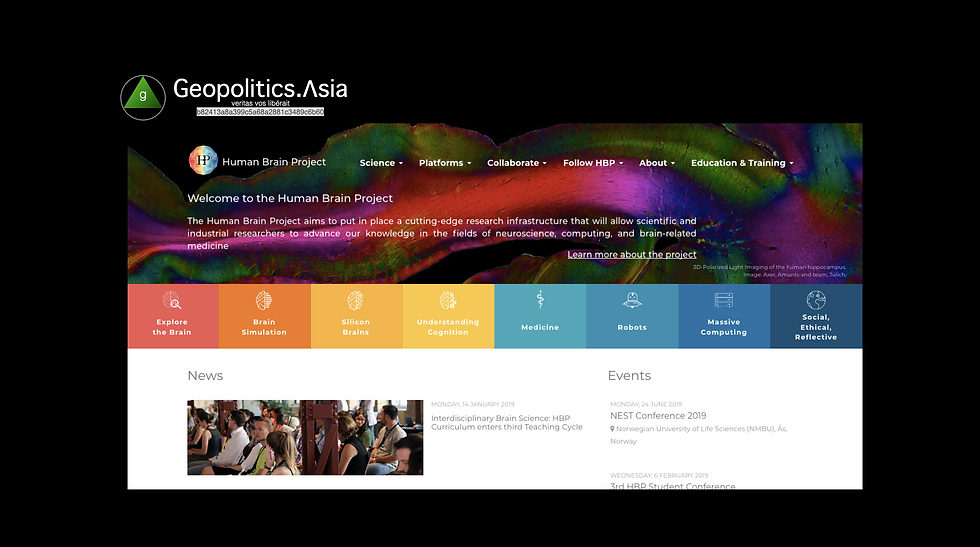
We should think about "Brain study program" like this HBP and Brain Initiative as an advanced research program in cognitive enhancement project. The more abstract research project can be found in NBIC project like Managing Nano-Bio-Info-Cogno: Innovations Converging Technologies in Society
Level 2: AI Applications and Basic Data Manipulation: This level introduces learners to various AI applications such as chatbots (like ChatGPT) and tools (like Midjourney). The primary focus is on understanding different AI models and their uses, such as generative AI, text-to-speech, etc. Essential skills in libraries like Numpy, Pandas, and Matplotlib for data manipulation and analysis also form part of this level.
Level 3: Introduction to Machine Learning: This level equips learners with an understanding of basic machine learning concepts and algorithms. The learning process allows comfort with Scikit-Learn libraries and developing an understanding of vital concepts like regression, classification, clustering, etc.
Level 4: Advanced Machine Learning, Statistics, and Advanced AI Applications: In this level, learners deepen their understanding of various machine learning models and ensemble methods. It also solidifies the understanding of statistics. Further exploration of advanced AI applications and tools are also undertaken.
Level 5: Introduction to Deep Learning, Basic Prompting, and Introduction to Vector Databases: At this level, learners acquire skills in understanding and implementing deep learning models using libraries like TensorFlow or PyTorch. They deal with concepts like CNNs, RNNs, LSTM, etc. There is also an introduction to the concept of prompts in AI, especially in language models, and an initial exposure to vector databases and handling high-dimensional data.
Level 6: Advanced Deep Learning, NLP, Advanced Prompting, and Vector Databases:This level offers learners more understanding regarding complex architectures like transformers and attention mechanisms. They will delve into NLP and models like BERT, GPT-2, etc. Learners also develop an understanding of different prompting techniques, such as one-shot, few-shot learning, chain of thought, and tree of thought. Furthermore, they enhance their understanding of vector databases and related search mechanisms like KNN.
Level 7: Building ML Models from Scratch, Deploying ML Models, and Proficiency in Vector Databases: This level requires proficiency in taking a model from development to deployment and understanding MLOps concepts. Learners can build custom models, including large language models, and gain knowledge about pre-training and fine-tuning. There is further exploration of advanced prompting techniques in practical applications. At this level, learners should also be proficient in utilizing vector databases and managing high-dimensional data.
Level 8: Autonomous AI, Custom Language Models, and Advanced LLM Creation:This advanced level focuses on autonomous AI agents, building and deploying custom language models, and utilizing libraries like LangChain. Learners need to have a mastery of advanced prompting techniques and understanding how to build advanced language models like GPT-3.5 or GPT-4 from scratch.
Level 9: Quantum Computing and AI Research: This curriculum now takes learners into advanced research in quantum computing and AI, focusing on the proficiency in Quantum Neural Networks and ability to publish in academic journals.
Level 10: AI Leadership and Innovation: This final level is reserved for individuals who are not just highly proficient with advanced AI technologies and techniques, but they're also leading and innovating within the field. It may involve designing new AI architectures, pioneering novel applications of AI, shaping AI policy and ethics, influencing education in AI, or making significant contributions to AI research that push the boundaries of what's currently possible.
Discussion: Model Fine-Tuning
Model fine-tuning, as suggested in GPT-3.5 Turbo fine-tuning, is a cost-effective form of transfer learning. It involves repurposing a pre-trained model for a different task, without the necessity of extensive training from scratch. For example, if an object-detection model is developed to identify cars, it can be fine-tuned for a similar task such as identifying trucks, by using the model's understanding of common features like headlights and wheels. For us, we expect to do a fine-tuning on metageopolitics. In essence, fine-tuning involves making slight modifications to the existing model without a complete overhaul.
To pursue a knowledge on above suggested curriculum and model fine-tuning, we recommend to enroll with DeepLearning.ai and some webinar like this
One such method of fine-tuning is Parameter-Efficient Fine-Tuning, which employs techniques like Low-Rank Adaption (LoRA). LoRA is fast becoming an industry standard due to its ability to reduce costs and increase efficiency. Notably, it allows specific parts of a model to be fine-tuned rather than the entire model, enabling easy adaption to new datasets without constructing an entirely new model. The computational efficiency relies on its ability to reduce a large number of computations.
Through fine-tuning, organizations can adapt various cutting-edge models for their specific business needs. Stable Fusion, an image generation model that can be directed using natural language prompts, Whisper AI, a speech-to-text transcribing model, and Large Language Model Meta AI (LLaMA), used for text-to-text applications, are some models that can be tailored to deliver specific solutions.
Comments