In a breathtakingly evocative scene that harks back to the dawn of mankind, the opening act of the iconic movie 2001: A Space Odyssey offers an enigmatic glimpse into the genesis of a transformative encounter. This moment, as shrouded in mystery as it is rich in symbolism, features a mirror-like monolith rising majestically amidst a desert bathed in hues of light blue and pink. The photorealistic depiction of this surreal landscape bears a stark resemblance to the ever-evolving world of artificial intelligence, where the line between reality and digital art continues to blur. Just as the enigmatic monolith triggers a dramatic shift in the ape's evolutionary trajectory, the relentless advancement of AI promises to profoundly reshape our world, instilling a sense of excitement, exploration, and unwavering confidence in our collective future.
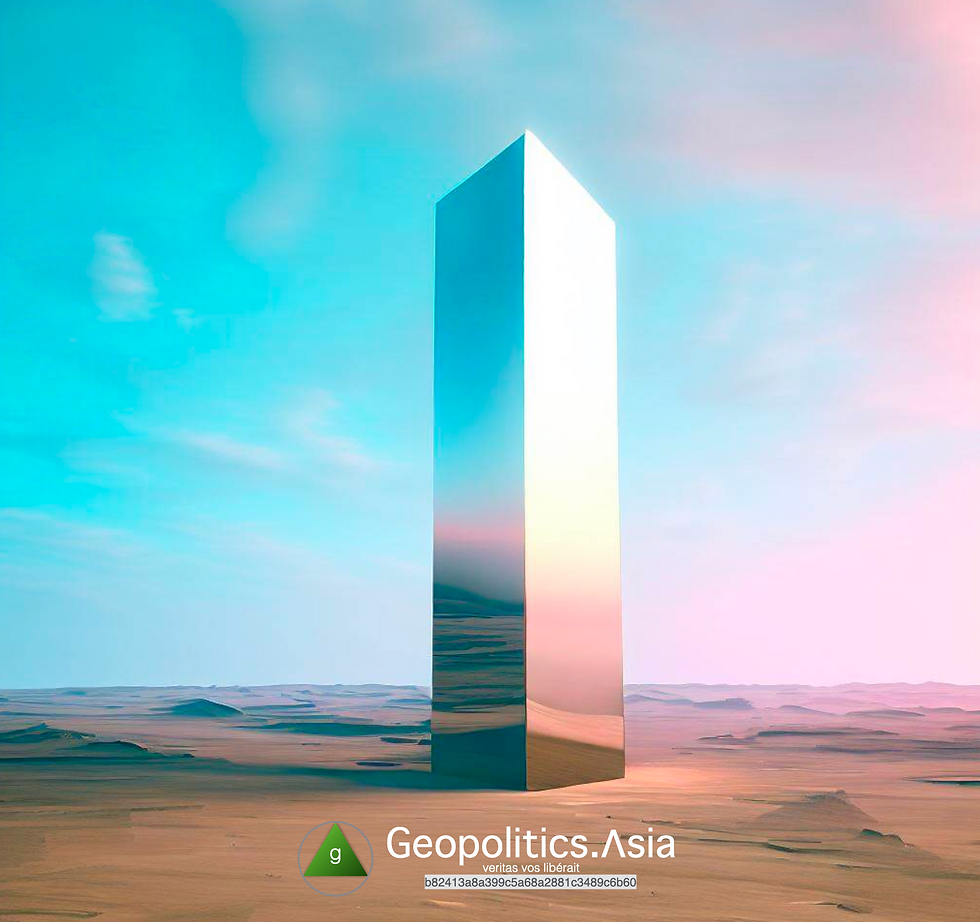
Image created by Bing, by "surprise me" prompt
On March 15th, 2022, OpenAI released new versions of its API models, GPT-3 and Codex, which included edit and insert capabilities. These models were named "text-davinci-003" and "code-davinci-002" respectively. OpenAI touted them as more capable than their predecessors, having been trained on data up to June 2021.
Later, on November 30th, 2022, OpenAI announced the release of the GPT-3.5 series, which included ChatGPT, a model fine-tuned from a GPT-3.5 model. This marked a significant transition in the evolution of the OpenAI API, a moment we call the "Epochal Era Escapade".
Today, 119 days after the Epochal Era Escapade, apart of O'Reilly's warning of the red-hot summer of AI, we continue to see important developments, such as the partnership between Replit and Google Cloud. Replit is an online Integrated Development Environment (IDE) that focuses on providing a collaborative coding platform with seamless GitHub integration. On the other hand, Visual Studio is a comprehensive desktop IDE with a wide range of tools and functionalities for software development. This partnership represents the first impactful counter from Google against Microsoft since Google's controversial demo of the Chatbot Bart last month.
Last week, NVIDIA, a key technology company in the AI industry spanning across hardware, software, and ecosystem development, announced significant updates during the GPU Technology Conference (GTC) 2023. As a pioneer in developing Graphics Processing Units (GPUs), NVIDIA's GPUs are essential for powering AI applications. Their capability to handle massive amounts of data and parallel processing makes them ideal for AI workloads such as deep learning and neural network training.
The key issues NVIDIA announced during the conference are:
Accessibility: NVIDIA aims to make its AI products more accessible, focusing on generative AI applications and enterprise-grade cloud-delivered AI infrastructure.
AI inference platforms: NVIDIA has released three computing platforms for AI inferencing - DGX H100, NVIDIA L4, and H100 NVL. Early adopters include Microsoft and Google Cloud.
DGX Cloud: NVIDIA has taken its DGX AI supercomputing service to the cloud, making AI supercomputing infrastructure more accessible and cost-effective. DGX Cloud is currently available on Oracle Cloud and will be available on Microsoft Azure, Google Cloud, and others in the future.
NVIDIA cuLitho: NVIDIA developed a software library for computational lithography in collaboration with TSMC, ASML, and Synopsys, aiming to accelerate the design and manufacture of a new generation of chips.
Omniverse Cloud collaboration with Microsoft: NVIDIA Omniverse Cloud is a suite of cloud services for content creators, artists, and developers. Microsoft Azure has partnered with NVIDIA as the cloud service provider for Omniverse Cloud, integrating Microsoft 365 applications like Teams, OneDrive, and SharePoint on the platform. Early adopters include BMW Group, Geely Lotus, and Jaguar Land Rover.
The AI industry has reached significant milestones in recent times. For instance, Glass Health AI 2.0, which passed Medical Licensing Exams, GPT4All, a new 7B LLM based on LLaMa run locally on a notebook computer, ChatPDF, a chatbot that can analyze any PDF, and the announcement of fully open-source GPT-3 architecture models by Cerebras.
On the business front, there is a news report that a Wharton professor had AI chatbots work on a business project for 30 minutes. Bing wrote 1,757 words in under three minutes, and ChatGPT wrote code to build an entire website. Additionally, there is a story of a man who successfully asked ChatGPT to turn $100 into a business that could generate as much profit as possible.
In our previous article, we posed the question not of whether to adopt an AI-driven business strategy, but rather how and how soon to adopt an AI-tensibility strategy with Adaptive Team Strategists as a core engine of AI-Driven, Agile Organizational Models. The key measure of the advantage of modern AI-Driven, Agile Organizational Models over traditional non or low adoption rates of AI-Driven business can be gauged by lower costs, lower labor, and higher profits. Alternatively, a strategy that prioritizes quality over quantity can produce exponential output with high quality that traditional companies before the "Epochal Era Escapade" (or pre-AI era date) on November 30th, 2022, could never have dreamed of catching up with.
As we highlighted earlier, one of the core functions of the Adaptive Team Strategist is to leverage technology and data-driven insights. This includes being proficient in using AI and other advanced technologies to make informed decisions and improve team performance. They must stay informed about technological advancements, train team members to use these tools effectively, and incorporate data-driven insights into the decision-making process.
In this week's trend monitoring article, we'll delve into the history of AI technology, particularly Generative AI (GAI)/AI-Generated Content (AIGC), the bread and butter of modern AI-Driven, Agile Organizational Models. Understanding the history of AI technology is crucial to understanding its current capabilities and potential for the future.
History of AI and AGI
As shown in the "Category of AI technologies" table above, AI can be categorized according to technologies or functions. AI is often used in specific duties, such as in web applications like Facebook, Twitter, Instagram, YouTube, or Amazon's digital bookstore, where AI is used to identify the audience's preferred items statistically, increasing engagement on the website or application. However, generative AI, such as OpenAI's GPT, is different. It has been built with super-high ambitions, aiming to achieve Artificial General Intelligence (AGI).
The concept of "Artificial General Intelligence" (AGI,) also known as strong AI or general AI, can be traced back to the early days of artificial intelligence research. Although it is difficult to attribute the idea to a single individual, mathematician and computer scientist Alan Turing can be considered one of the pioneers in this field. In 1950, he introduced the Turing Test, which aimed to determine whether a machine could exhibit intelligent behavior indistinguishable from that of a human.
The term AGI was coined later by John McCarthy, Marvin Minsky, Nathaniel Rochester, and Claude Shannon, who proposed at the Dartmouth Conference in 1956 that "every aspect of learning or any other feature of intelligence can, in principle, be so precisely described that a machine can be made to simulate it." This statement laid the foundation for the development of AGI, although the term was not explicitly used at the time.
The explicit use of the term AGI is more recent and often credited to Shane Legg and Ben Goertzel, who co-founded the Artificial General Intelligence Research Institute (AGIRI) in 2001, which later evolved into OpenCog, an open-source software project aimed at creating AGI. The term AGI has been widely adopted in the AI research community since then to describe machines with human-like cognitive abilities across various tasks and domains.
AGI is very important because, unlike other AI technologies, genuine AGI is capable of writing code to revise itself, similar to carbon-based life forms that can change through genetic mechanisms over generations, or humans who use "culture" and "language" to change course of themselves. However, AGI's self-revision in coding is much faster and more efficient. AGI can be a command center of other AIs.
GPT, a general purpose AI and a stepping stone for AGI, is considered a Large Language Model (LLM) that uses Transformer Models as its core architecture. The Transformer architecture is particularly well-suited for processing and generating large-scale text data, making it the foundation for many LLMs, including GPT-3, GPT-4, BERT, T5, and others. These LLMs, built upon the Transformer architecture, have demonstrated strong performance in various natural language processing tasks, such as text generation, translation, summarization, question-answering, and sentiment analysis.
The Formation of OpenAI and GPT
In December 2015, Sam Altman, Greg Brockman, Reid Hoffman, Jessica Livingston, Peter Thiel, Elon Musk, Amazon Web Services (AWS), Infosys, and YC Research announced the formation of OpenAI, pledging over $1 billion to the venture. The organization, headquartered at the Pioneer Building in San Francisco's Mission District, committed to "freely collaborate" with other institutions and researchers by making its patents and research open to the public. According to Wired, Brockman met with Yoshua Bengio, a deep learning pioneer, and created a list of the best researchers in the field. In December 2015, Brockman successfully hired nine of them as OpenAI's first employees. Although the organization paid corporate-level salaries in 2016, AI researchers at OpenAI did not receive salaries comparable to those at Facebook or Google.
The paper "Attention is All You Need" is a groundbreaking research paper in the field of natural language processing and machine learning, written by a team of researchers from Google Brain. The authors of the paper include Ashish Vaswani, Noam Shazeer, Niki Parmar, Jakob Uszkoreit, Llion Jones, Aidan N. Gomez, Łukasz Kaiser, and Illia Polosukhin.
Published in 2017, the paper introduces the Transformer architecture, a novel neural network architecture that relies on self-attention mechanisms instead of recurrent or convolutional layers to process input data. This attention-based approach allows the model to process and learn from long-range dependencies in the input sequence more effectively than previous architectures, such as RNNs and LSTMs.
The Transformer architecture has since become the foundation for many state-of-the-art LLMs, including BERT (Bidirectional Encoder Representations from Transformers), GPT (Generative Pre-trained Transformer), and T5 (Text-to-Text Transfer Transformer), among others. These models have significantly advanced the performance of a wide range of natural language processing tasks, such as machine translation, text summarization, sentiment analysis, and question-answering.
GPT and Reinforcement Learning from Human Feedback (RLHF)
Generative Pre-trained Transformer 2 (GPT-2) is an open-source artificial intelligence model developed by OpenAI in February 2019. GPT-2 is capable of translating text, answering questions, summarizing passages, and generating human-like text output, although it may become repetitive or nonsensical in longer passages. As a general-purpose learner, GPT-2's ability to perform various tasks stems from its capacity to accurately synthesize the next item in an arbitrary sequence.
The GPT-2 model is a ten-fold scale-up from OpenAI's 2018 GPT model in terms of parameter count and training dataset size. It utilizes a deep neural network, specifically a transformer model with attention mechanisms, allowing it to selectively focus on relevant segments of input text and enabling increased parallelization. This approach outperforms previous RNN/CNN/LSTM-based models. OpenAI released the complete 1.5 billion parameter GPT-2 model in November 2019, followed by the 175-billion-parameter GPT-3 in 2020. However, GPT-3's source code has not been made public, with access provided exclusively through APIs offered by OpenAI and Microsoft.
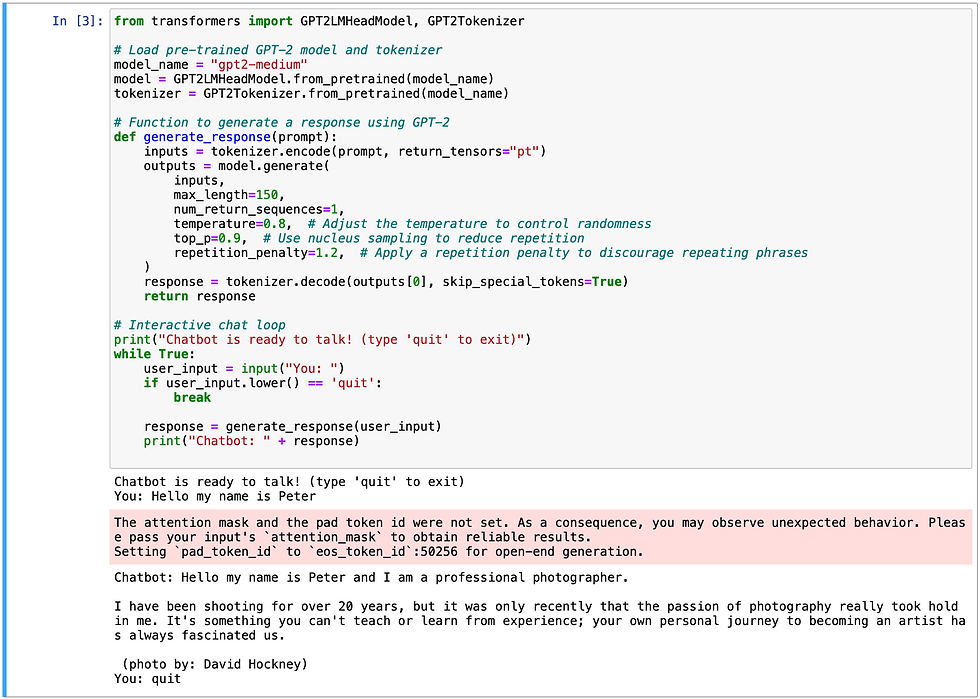
The responses from the pre-trained GPT-2 (Hugging Face Transformers) are irrelevant to the 'question,' although they are readable, see this code at GitHub
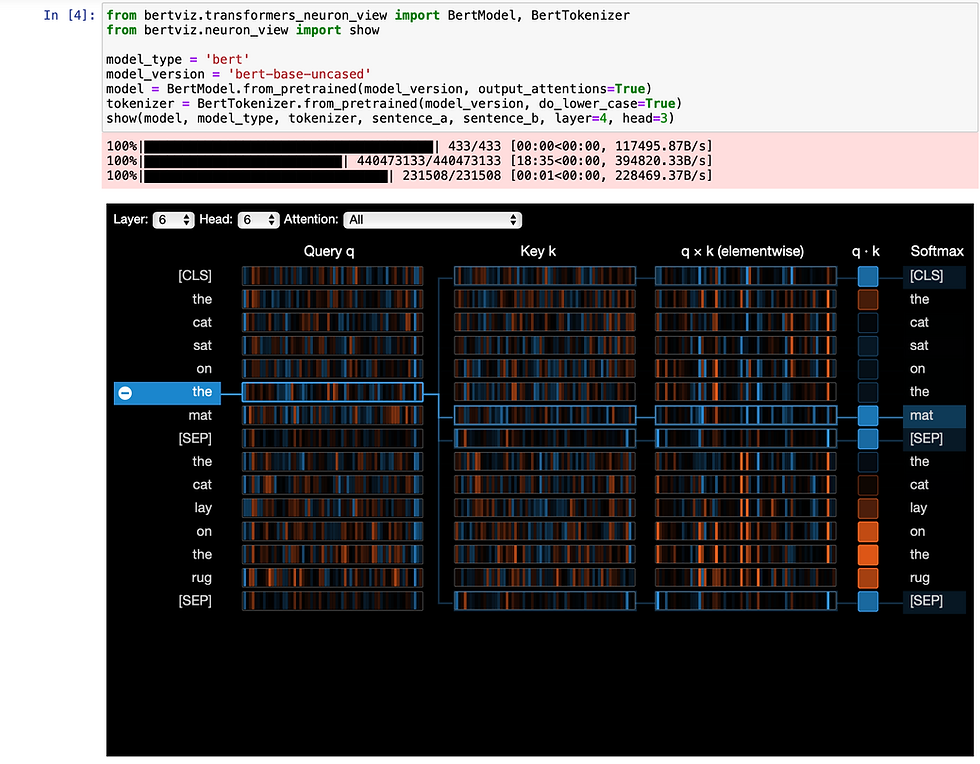
We can employ a handy library called BertViz for Jupyter to visualize the calculation of attention weights. This library offers numerous functions that facilitate the visualization of various attention aspects in transformer models. To display the attention weights, we can utilize the neuron_view module, which tracks the computation of the weights, illustrating the integration of query and key vectors to generate the final weight. See code at GitHub, and O'Reilly Media's book, "Natural Language Processing with Transformers, Revised Edition". Running BertViz may consume huge resource, please consider testing at colab.
The GPT-2 repository provides various scripts and files that enable users to work with the model. These include encoder.py, generate_unconditional_samples.py, interactive_conditional_samples.py, model.py, and sample.py. The pre-trained GPT-2 model is also provided in the repository, which can be used for various natural language processing tasks without needing to train it from scratch.
However, users can fine-tune the pre-trained GPT-2 model on a specific dataset to better suit a particular use case or domain. Fine-tuning is a process that involves training the model for additional iterations on new data to refine its understanding of the problem domain.
The Hugging Face Transformers library provides an easy-to-use interface for working with various transformer models, including GPT-2. To fine-tune GPT-2, users need to install the Transformers library using pip and prepare a suitable dataset. Tokenizing the dataset using the GPT-2 tokenizer from the Transformers library is the next step. Then, users can load the pre-trained GPT-2 model and tokenizer, configure the training settings, and fine-tune the model using the Trainer class from the Transformers library. Fine-tuning GPT-2 requires considerable computational resources, and using a GPU or TPU is recommended for faster training.

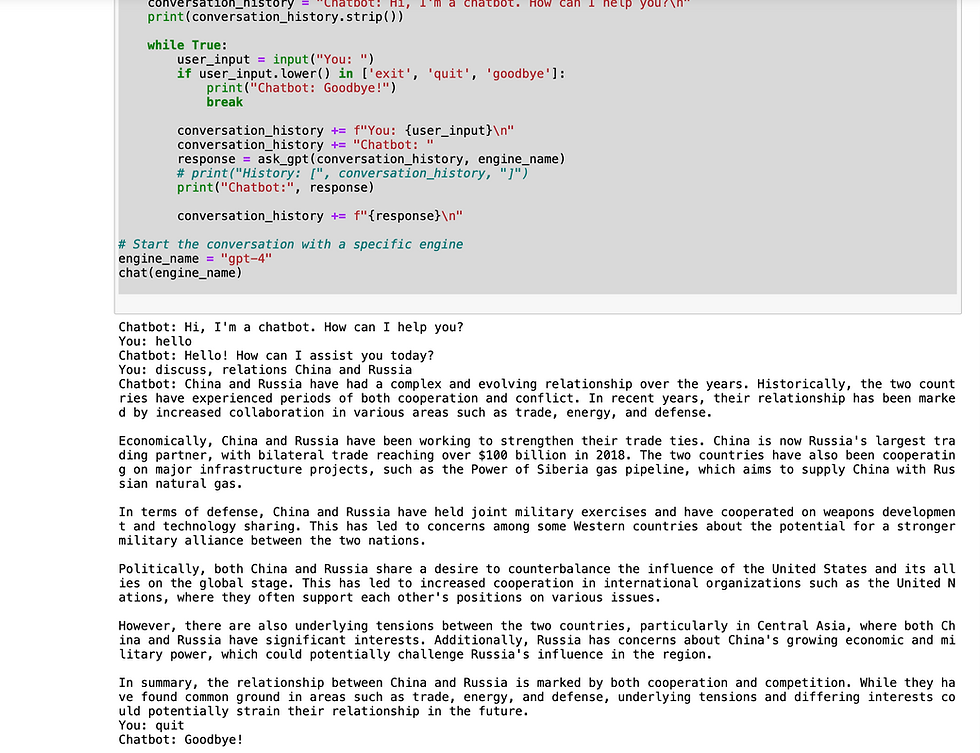
Chatbots developed with the GPT-4 API are much more efficient and provide relevant information according to the question (prompt). The GPT-4 model can consume more data and has 4x the context length (32,768 tokens). Similar to ChatGPT, this Chatbot can accumulate the previous conversation records as the "context", please see code at GitHub. You can get access GPT-4 API via invitation from OpenAI. Chatbot is a prime example on GTP's application to exploit RLHF as best and naturally.
Reinforcement Learning from Human Feedback (RLHF) is a machine learning technique that trains a reward model using human feedback to optimize an agent's policy through reinforcement learning (RL). This method enhances the robustness and exploration of RL agents, particularly when the reward function is sparse or noisy. Human feedback is gathered by ranking the agent's behavior, which can be used to score outputs using systems such as the Elo rating system. RLHF has been applied to various natural language processing domains, including conversational agents, text summarization, and natural language understanding. Traditional reinforcement learning, which relies on a reward function, is challenging to apply to these tasks, as rewards are often difficult to define or measure, especially in complex tasks involving human values or preferences. RLHF enables language models to produce answers that align with complex values, generate more verbose responses, and reject inappropriate or out-of-scope questions. Examples of RLHF-trained language models include OpenAI's ChatGPT and InstructGPT, as well as DeepMind's Sparrow.
Given the fast pace of development in the field of generative AI, the latest models (with helps from RLHF) are now capable of outperforming several test and ground truth (GT) assessments. This is a testament to the underlying technology and its ability to continually push the boundaries of what's possible in natural language processing. As generative AI continues to evolve and mature, we can expect even more impressive advancements in the future.
Conclusion
As we stand on the precipice of a new era, the rise of artificial intelligence, akin to the miraculous appearance of the mirror-like monolith, ushers in a future of unprecedented potential. Endowed with the ability to augment human cognition to seemingly superhuman levels, AI has the power to elevate our collective capabilities and redefine the boundaries of possibility. With bated breath, we embark on a thrilling odyssey to explore the vast, uncharted territories that lie ahead, where the confluence of human intellect and machine learning will lead to groundbreaking discoveries and unimaginable innovation. In this exhilarating new world, we will harness the transformative power of AI to forge a bold, fearless, and confident future, transcending the limits of what we once thought possible.
Geopolitics.Asia will provide serious policy analysis on Mondays, trend monitoring on weekdays, and cultural and lifestyle issues on weekends. Please note that our weekday situation monitoring will not include a trend radar or scenario analysis for the time being, as we work to fully automate these processes with AI. You can, however, access to our previous experiments on trend radar and scenario planning generated by the AI, 1) Simple scenario planning at Jan 26, 2023, 2) Double iteration scenario planning technique at February 2, 2023, 3) Triple iteration scenario planning technique at February 9, 2023, and 4) Hyperdimensional scenario planning technique at February 17, 2023.
Stay tuned for updates on this exciting development!
Comments