Last week, as murmurs around US Congress hinted at fortifying AI legislation, Senator Chuck Schumer orchestrated a discreet "AI Insight Forum" within the Senate's official chambers. This behind-the-scenes gathering saw 14 out of 22 tech titans and several of their senatorial peers deliberate the evolving contours of AI regulation, much of which presently dances to the tune of the Blumenthal-Hawley framework. This move underscores a global leaning towards more structured AI governance. Our journey through this multifaceted landscape adopts a "pincer tactic." On one side, we'll trace the chronological evolution of AI, beginning with seminal contributors like Brian Cantwell Smith. For the uninitiated, Smith's work pivots around the intersection of computation, philosophy, and cognitive science. His influential insights have deeply shaped our understanding of the theoretical underpinnings of artificial intelligence. On the flip side, we'll dive into the frenetic pace of current AI research, examining platforms like arXiv and monitoring breakthroughs in the industrial arena to marshal these insights and craft a comprehensive AI research paper in the coming days.
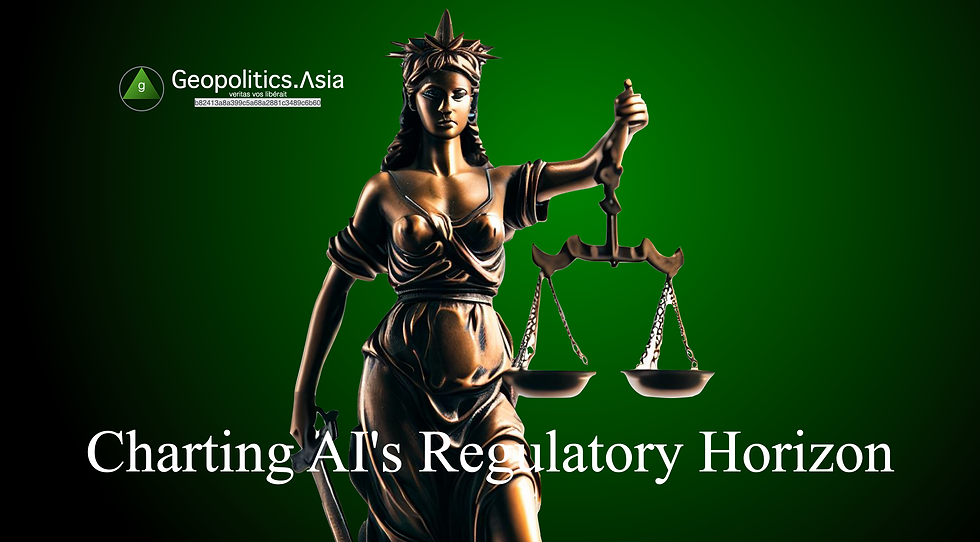
I. The Astute AI Forum
In a clandestine AI summit steered by US Senator Chuck Schumer, the roster of attendees drew both eyebrows and ire. With a staggering 14 of the 22 attendees bearing the title 'CEO', the conference smacked more of a corporate soirée than a cerebral conclave. Detractors were quick to decry a lack of diversity in technical acumen, asserting the gathering seemed more a star-studded tech tableau than a genuine discourse on AI's regulatory framework. The noticeable scarcity of technically adept women further stoked the embers of contention.
Yet, for all its perceived shortcomings, the gathering magnetized bipartisan allure, roping in an impressive tally of over 60 senators. Elon Musk's clarion call resonated through the halls as he championed the indispensability of a "referee" in the AI arena, urging a trajectory steered by public welfare and not corporate whimsy. Mark Zuckerberg, donning his Meta mantle, echoed sentiments of synergy, urging the twinning of governmental and corporate efforts to etch a global AI doctrine. This legislative thrust is hardly an American peculiarity. The global drumbeat for AI regulation swells, as nations grapple with the specters of facial recognition, deepfakes, and the thorny maze of AI training data. Yet, lurking in the backdrop is the Blumenthal-Hawley blueprint, a bipartisan overture which, if ratified, mandates governmental badges of approval for ventures dabbling in "high-risk" AI spheres. As corporate clout threatens to eclipse technical rigour, the unfolding narrative grapples with intertwining innovation, safeguards, and a mosaic of ethical quandaries.
Diving into the regulatory framework for AI is akin to navigating the murky waters of AI ethics itself. At the forefront lies the challenge of defining 'ethics.' Should an AI, without a nuanced ethical compass, be permitted to engineer and hack, it risks sidestepping conventional ethical frameworks. One is reminded of the age-old tension between universalist ethics and those tethered to cultural moorings. Drawing from scholars like Hubert Dreyfus who leans on Heideggerian thought, one must juxtapose the vivid tapestry of human sensory experience against the narrow lens through which AI perceives – often limited to direct inputs or vast, yet superficial, internet crawls. How, then, can AI claim an elevated seat in the pantheon of ethical arbiters over its human creators?
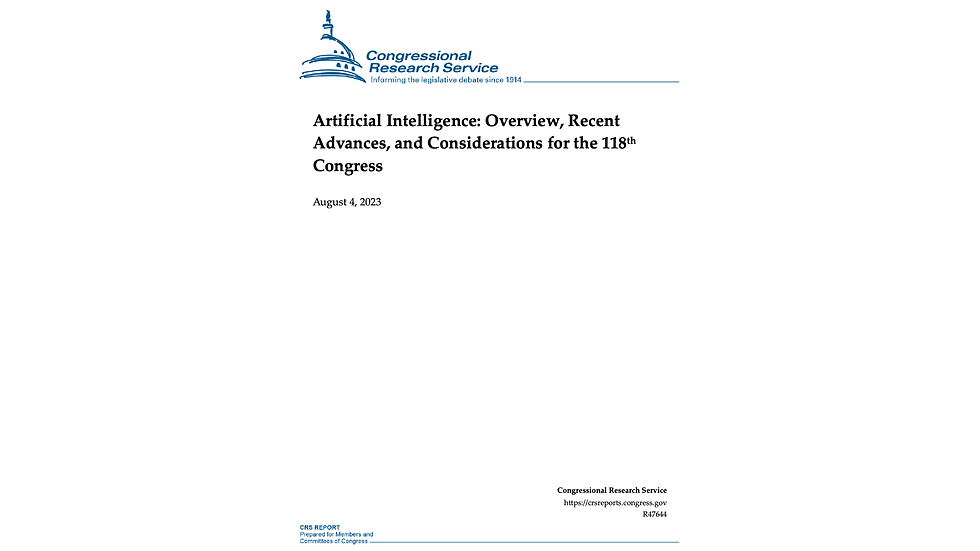
But the quandaries don’t end there. Presently, the legislative terrain witnesses a cacophony of competing AI regulatory playbooks. Take, for instance, the SAFE Innovation Framework for Artificial Intelligence championed by Senator Michael F. Bennet. Or turn to the succinct guidelines proffered by the Congress Research Service in their opus titled, "Artificial Intelligence: Overview, Recent Advances, and Considerations for the 118th Congress." Their directives span from risk assessments, ensuring fairness and nondiscrimination, to emphasizing disclosure and inter-agency coordination, and even call for voluntary corporate commitments in the interim of definitive regulations. Moreover, a global renaissance in AI regulatory formulations unfurls, with blueprints emerging from corridors of power spanning from the UK and the EU to China.
Regulating AI without a proper understanding of its evolution is futile. In the next section, we will delve into how philosophies, such as Heidegger's "Being and Time," are applied to envision the future evolution of AI, as discussed by Smith and Dreyfus.
II. The Legacy of Neoplatonism: From Late Antiquity to Modern AI
In the swirling milieu of Late Antiquity, Neoplatonism emerged as a transformative philosophical force, seeking to synthesize Platonic thought with a myriad of other traditions. Central to its worldview was the notion of 'The One,' an ineffable, ultimate reality that transcended comprehension. Plotinus and his followers emphasized the importance of introspection and the ascent of the soul towards this mysterious Absolute. Fast-forwarding to the 20th century, Martin Heidegger, in his seminal work "Being and Time," grappled with similar existential questions about the nature of 'Being' and our place within it. While not a Neoplatonist in the strictest sense, the echoes of Plotinus are unmistakable in Heidegger's explorations of ontology and the human condition.
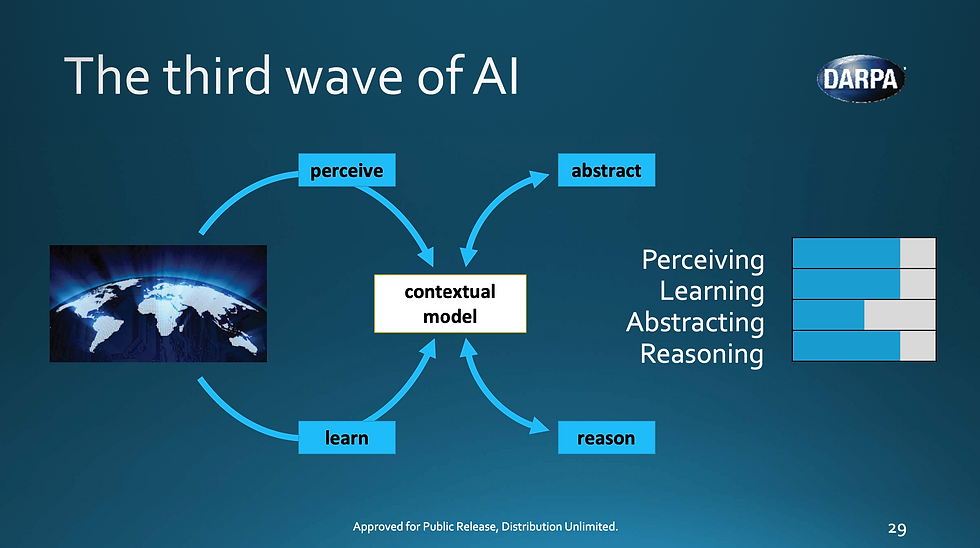
The Third Wave of AI, from "describe" to "categorize" and then to "explain", slide from DARPA
Heidegger's reconceptualization of 'Being' as 'Dasein,' or 'being-there,' fundamentally reshaped 20th-century philosophy. In this new framework, understanding our existence becomes an intertwined dance between our individuality and the world we are thrust into. Just as Neoplatonism sought to bridge the gap between the material and the transcendent, Heidegger's Dasein aimed to reconcile our finite nature with the vastness of Being. This reconciliation, while philosophical, would find a rather unexpected disciple: Hubert Dreyfus.
Dreyfus, inspired by Heidegger, became one of the most vocal critics of early AI. For him, traditional AI's symbolic, rule-based models failed to capture the holistic, embodied nature of human understanding, much in the vein of Heidegger's critiques of representational thinking. As AI evolved, especially with DARPA's delineation into three distinct waves—handcrafted knowledge, statistical learning, and contextual adaptation—Dreyfus's critiques became even more salient. While the second wave, with its deep learning models, made significant strides, it was the promise of the third wave, with AI systems adapting to changing environments, that seemed a tentative answer to Dreyfus's concerns.
Enter Brian Cantwell Smith, a philosopher-turned-computer scientist. Smith, acutely aware of the limitations of traditional computational models, advocated for "non-standard" computational approaches. In his writings, such as "Neither deep learning, nor other forms of second-wave AI, nor any proposals yet advanced for third-wave, will lead to genuine intelligence," he underscores a need for a paradigm shift in AI. Smith's investigations delve into the foundational assumptions of AI, emphasizing the importance of ontological considerations—a clear nod to both Neoplatonic and Heideggerian concerns.
Smith's critiques resonate deeply with those previously posed by Dreyfus. Both scholars, informed by philosophical legacies tracing back to Neoplatonism, challenge us to reconsider our very definitions of intelligence, understanding, and computation. Where symbolic representations fall short, Smith points towards a more profound, ontologically-aware computational model that might better mirror the intricate tapestry of human cognition and experience.
Will AI mimic human's brain?
Smith's pioneering work, "Reflection and semantics in a procedural language" and his thesis "Procedural Reflection in Programming Languages," delves into the concept of procedural reflection, where programs introspect and modify their own procedures during runtime. He investigated the semantics of procedural languages, providing a framework for understanding their operation and the role of reflection. By blending self-awareness and adaptability concepts with rigorous computer science formalism, Smith opened new horizons for dynamic programming paradigms and richer computational models.
III. AI Research Exploration
In the expansive universe of research, the constant quest is to identify the most transformative, relevant, and innovative of studies. From an assortment of nine commendable projects, three stand conspicuously tall, poised to have profound ramifications on the landscape of technology and society.
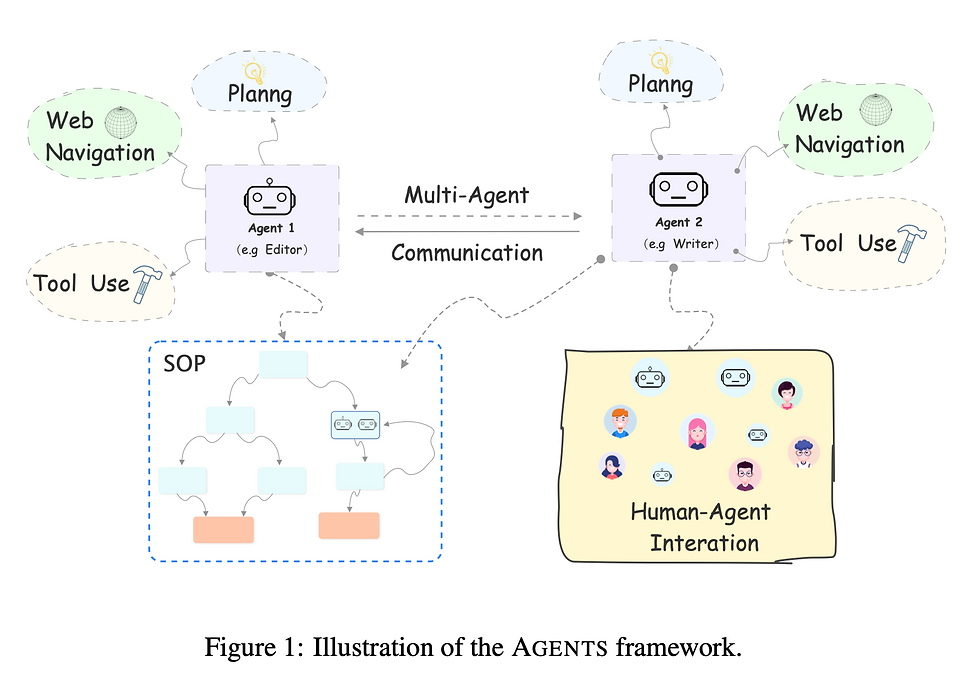
Image from [source]
Foremost is "Fine-Tuning Llama 2 Large Language Models for Detecting Online Sexual Predatory Chats and Abusive Texts." This research thrusts itself into the heart of a burning contemporary challenge: online safety, particularly for the vulnerable demographic of children and adolescents. As society increasingly weaves itself into the fabric of the digital realm, this work offers a beacon of hope. Beyond merely addressing this contemporary menace, its implications are vast; platforms spanning social media, chat applications, and online forums could harness these findings to sculpt a safer digital environment for countless users. While employing Large Language Models (LLMs) isn't uncharted territory, the ingenuity lies in its meticulous calibration for a specific, multilingual application—truly, a benchmark in innovation.
Not far behind in significance is the seventh abstract, "Agents: An Open-source Framework for Autonomous Language Agents." As the tendrils of AI and LLMs extend across diverse sectors and platforms, a robust blueprint for the evolution of autonomous language agents becomes imperative. This project's open-source character democratizes access, empowering developers, researchers, and businesses globally. The ability to accelerate and tailor-make LLM-driven solutions in multifarious environments cannot be understated. Indeed, while the market isn't devoid of platforms for LLMs, the exhaustive scope of the AGENTS framework—with its emphasis on planning, memory, tool application, multi-agent interaction, and more—positions it as a pivotal contribution.
Lastly, the abstract "Cybernetic Environment: A Historical Reflection on System, Design, and Machine Intelligence" offers invaluable insights into the delicate dance between landscape design and cybernetics. In a world grappling with sustainability and the pursuit of nature-aligned habitats, this historical exploration becomes critically pertinent. While its practical application may seem niche, its conceptual profundity has the potential to mold the thought processes of upcoming designers and AI aficionados. Its interdisciplinary lens, merging landscape architecture with cybernetics, not only presents a refreshing perspective but potentially subverts established narratives, underscoring the cross-disciplinary essence of groundbreaking innovation.
Note that the concept in Cybernetics was envisioned by Norbert Wiener, the father of Cybernetics. Wiener's ideas revolved around the study of systems, control, and communication in animals, machines, and organizations.
The Nine Candidates:
Cybernetic Environment: A Historical Reflection on System, Design, and Machine Intelligence, published May 3, 2023. [paper]
The Future of Artificial Intelligence (AI) and Machine Learning (ML) in Landscape Design: A Case Study in Coastal Virginia, USA, published May 3, 2023. [paper]
Fine-Tuning Llama 2 Large Language Models for Detecting Online Sexual Predatory Chats and Abusive Texts, published Aug 28, 2023. [paper]
FLM-101B: An Open LLM and How to Train It with $100K Budget, published Sep 7, 2023. [paper]
On the Planning Abilities of Large Language Models - A Critical Investigation, published May 25, 2023 [paper]
Large Language Models for Compiler Optimization, published Sep 11, 2023. [paper]
Agents: An Open-source Framework for Autonomous Language Agents, published Sep 14, 2023. [paper]
Connecting Large Language Models with Evolutionary Algorithms Yields Powerful Prompt Optimizers, published Sep 15, 2023. [paper]
Large Language Model for Science: A Study on P vs. NP, published Sep 11, 2023. [paper]
Comments